
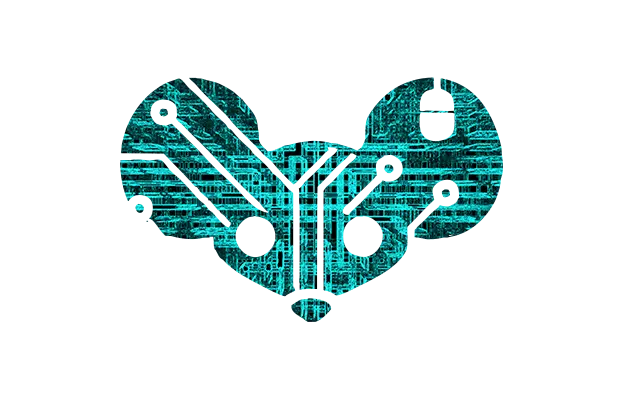
The general framework for evolutionary methods/genetic algorithms is indeed old but it’s extremely broad. What matters is how you actually mutate the algorithm being run given feedback. In this case, they’re using the same framework as genetic algorithms (iteratively building up solutions by repeatedly modifying an existing attempt after receiving feedback) but they use an LLM for two things:
-
Overall better sampling (the LLM has better heuristics for figuring out what to fix compared to handwritten techniques), meaning higher efficiency at finding a working solution.
-
“Open set” mutations: you don’t need to pre-define what changes can be made to the solution. The LLM can generate arbitrary mutations instead. In particular, AlphaEvolve can modify entire codebases as mutations, whereas prior work only modified single functions.
The “Related Work” (section 5) section of their whitepaper is probably what you’re looking for, see here.
Yep, since this is using Gaussian Splatting you’ll need multiple camera views and an initial point cloud. You get both for free from video via COLMAP.